Diabetic Retinography
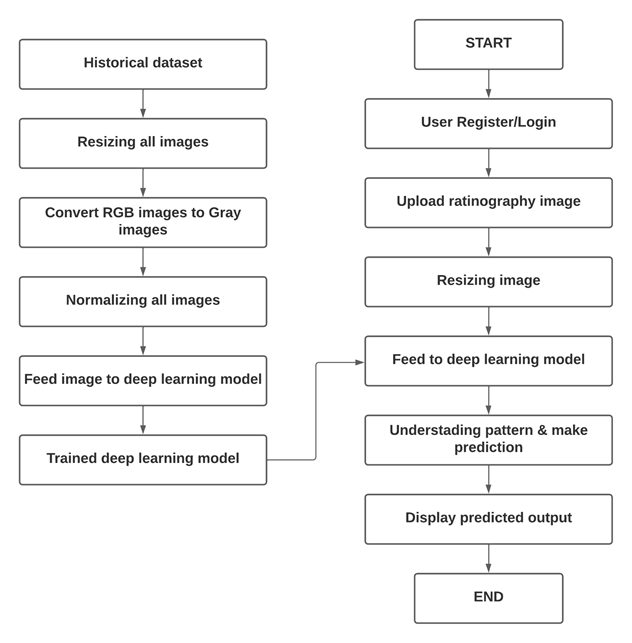
ABSTRACT: –
Diabetic retinopathy may be a common complication of diabetes mellitus that causes lesions on the tissue layer that have an effect on vision. If not detected in time, it will cause vision defects. Unfortunately, diabetic retinography isn’t a process and treatment solely preserves vision. Early detection and treatment of diabetic retinography will considerably reduce the chance of vision loss. The manual diagnostic method of retinal structure images in diabetic retinography is time, effort, and valuable, and vulnerable to misdiagnosis, in contrast to computer-aided diagnostic systems. Diabetic retinopathy could be a complication of polygenic disorder that targets the eyes by damaging the retinal blood vessels. it’s initially asymptomatic or causes unsteady vision issues. because it becomes severe, it affects each eye and eventually causes partial or total vision loss. It primarily happens once blood sugar levels are out of control. Therefore, someone with diabetes mellitus is usually exposed to a high risk of acquiring this disease. Early detection will deter the chance of complete and permanent blindness. It therefore requires an efficient screening system. This work deals with deep learning methodology, specifically DenseNet-169 Dense-Connected Convolutional Network, that is used for early detection of diabetic retinopathy. Classifies bodily structure images based on severity levels as no diabetic retinography, mild, moderate, severe, and proliferative diabetic retinography. The datasets thought of are the detection of diabetic retinopathy that is obtained from Kaggle. The planned technique is carried out through completely different steps: information collection, pre-processing, augmentation and modeling. The main goal of this work is to develop a robust system for automatic detection of diabetic retinography. Recently, deep learning has become one of the most common techniques that has achieved higher performance in several fields, especially within the analysis and classification of medical pictures. Convolutional neural networks are widely used as a deep learning method in medical image analysis and are highly effective. For this text, ways for detecting and classifying diabetic retinography background color images using deep learning techniques were reviewed and analyzed. Moreover, out there diabetic retinography datasets were reviewed for retinal color fundus. difficult differences that need a lot of investigation are discussed.
SYSTEM:-
- Data Collection Module: This module is responsible for acquiring retinal images from various sources such as clinics, hospitals and scans.
- Data Preprocessing Module: This module preprocesses input images to normalize brightness, contrast and sharpness.
- Feature Extraction Module: This module removes related features such as the presence of exudate, bleeding, and microaneurysm from preliminary images.
- Model Selection Module: This module selects the appropriate machine learning for retinopathy detection based on performance criteria such as accuracy, sensitivity, specificity, and AUC. Some of the algorithms used for this task are convolutional neural networks (CNN) and support vector machines (SVM).
- Referral Module: This module offers referral recommendations to physicians based on the degree of retinopathy given in the image.
- Reporting and Analysis Module: This module provides reporting and analysis capabilities to help clinicians monitor diabetic retinopathy symptoms and identify areas for improvement.
- Integration Module: This module integrates with other systems and applications such as electronic health records (EHR), telehealth platforms and imaging software.
Overall, this diabetic retinopathy test can help doctors diagnose diabetic retinopathy timely and accurately, thereby improving patient outcomes and reducing medical costs.
PROPOSED SYSTEM:-
Diabetic retinopathy is one of the serious problems that has gripped the whole world. To gain the attention of various researchers in order to find the optimal solution for the early detection of this disease, which in turn leads to the prevention of premature vision fluctuations. Many studies have been carried out and are still ongoing in this area with the aim of making life easier for both doctors and patients. This section provides an overview of the many research papers in the field of diabetic retinopathy.
While many researches or works have been concerned with the use of machine learning or data mining approaches, a completely different approach has also entered the way of detecting diabetic retinopathy. We use quantitative approaches to identify new parameters for the detection of proliferative diabetic retinopathy. It is based on the hypothesis that the location, number and area of lesions can improve the retinopathy prognosis process. The methods used for this study were Subjects and Imaging Data, Ultrawide Field Image Lesion Segmentation, Quantitative Lesion Parameters and Statistical Analysis. Comparison of lesions was performed based on number of lesions, surface area of lesions, distance of lesions from the center of the ONH, and regression analysis. We provide an overview of different methodologies for diabetic retinopathy using the detection of hemorrhages, microaneurysms, exudates as well as blood vessels and experimentally analyze the different results obtained from these methodologies to provide an in-depth insight into ongoing research. We focus primarily on different types of preprocessing and segmentation techniques and detail a procedure for detecting diabetic retinopathy in the human eye consisting of a number of systems and classifiers. We propose a diagnostic method for diabetic retinography using machine learning, namely SVM and Texture functions. The texture features used were LTP (Local Ternary Pattern) and LESH (Local Energy-based Shape Histogram) which gave better results compared to Local Binary Pattern (LBP).
A few years ago, deep learning was the most popular approach among researchers for detection, prediction, forecasting and classification tasks in various fields. In the medical field, especially in diabetic retinopathy, it reveals many possibilities for the prevention of such a terrible disease. We automatically detected diabetic retinopathy using a deep learning approach. The obtained results were compared with a machine learning approach such as SVM, where better results were obtained. The main goal of this work is to build a stable and noise-compatible system for the detection of diabetic retinopathy. This work uses a deep learning methodology to detect diabetic retinopathy based on the degree of severity (no DR, mild, moderate, severe and proliferative DR). Many processes were performed before the images were delivered to the network. In this work, we trained two models: our proposed model and a regression model, and then compared the accuracies obtained by these two models. Although our proposed model performed better than the regression model.
MODULES:-
- Data Collection Module: This module is responsible for acquiring retinal images from various sources such as clinics, hospitals and scans.
- Data Preprocessing Module: This module preprocesses input images to normalize brightness, contrast and sharpness.
- Feature Extraction Module: This module removes related features such as the presence of exudate, bleeding, and microaneurysm from preliminary images.
- Model Selection Module: This module selects the appropriate machine learning for retinopathy detection based on performance criteria such as accuracy, sensitivity, specificity, and AUC. Some of the algorithms used for this task are convolutional neural networks (CNN) and support vector machines (SVM).
- Referral Module: This module offers referral recommendations to physicians based on the degree of retinopathy given in the image.
- Reporting and Analysis Module: This module provides reporting and analysis capabilities to help clinicians monitor diabetic retinopathy symptoms and identify areas for improvement.
- Integration Module: This module integrates with other systems and applications such as electronic health records (EHR), telehealth platforms and imaging software.
Overall, this diabetic retinopathy test can help doctors diagnose diabetic retinopathy timely and accurately, thereby improving patient outcomes and reducing medical costs.
APPLICATION:-
- User Authentication: The application authenticates users such as doctors, patients, and caregivers to provide secure access to patient information.
- Photo Upload: Users can upload retina photos to the app from their device or camera connected to the device.
- Image Processing: The application preprocesses the input images to normalize brightness, contrast and sharpness.
- Feature Extraction: This application removes related features such as the presence of exudate, bleeding, and microaneurysm from preliminary images.
- Model Selection: The application selects the appropriate machine learning model for retinopathy detection based on performance measures such as accuracy, sensitivity, specificity, and AUC.
- Preferred algorithms can be CNN and SVM.
- Model Training: The app trains a machine learning model to choose from signature images where each image is labeled with or without diabetic retinopathy.
- Real-Time Scoring: The app scores retina images in real time and uses machine learning models to assign a retinopathy grade to each image. The rating system is usually from 0 to 4; where 0 indicates no retinopathy and 4 indicates severe retinopathy.
- Warnings and Warnings: If high-level retinopathy is given to the image, the application generates a warning or warning to the doctor.
- Referral recommendations: The app provides referral recommendations to physicians based on the level of retinopathy shown in the image.
- Reporting and Analysis: The app provides reporting and analysis features to help physicians monitor diabetic retinopathy and identify areas for improvement.
- Integration: The application integrates with other systems and applications such as EHRs, telehealth platforms and imaging software.
This diabetic retinopathy app helps doctors and patients screen and diagnose diabetic retinopathy quickly and easily, improving patient outcomes and reducing medical costs.
HARDWARE AND SOFTWARE REQUIREMENTS:-
HARDWARE:-
· Processor: Intel Core i3 or more.
· RAM: 4GB or more.
· Hard disk: 250 GB or more.
SOFTWARE:-
- Operating System: Windows 10, 7, 8.
- python
- anaconda
- Spyder, Jupyter notebook, Flask.
- MYSQL