SKIN DISEASE AND DIABETES CLASSIFICATION
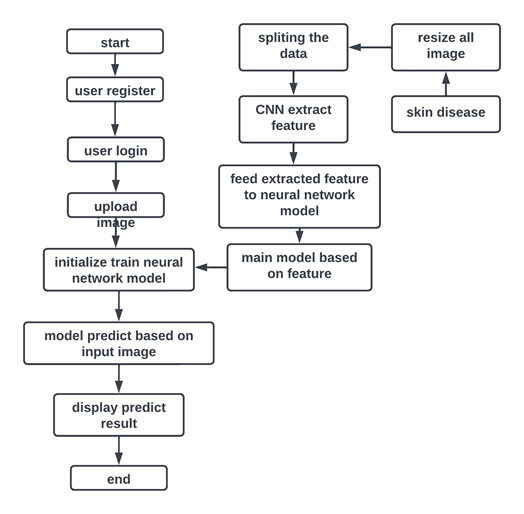
ABSTRACT:-
Dermatitis among humans has become a common disease, with millions suffering from various skin ailments. Often, these diseases have subtle dangers that not only lead to insecurity and depression but also lead to increased risk of skin cancer. Medical professionals and advanced instruments are needed to diagnose these skin diseases due to the lack of a visible solution in the images of skin diseases. The proposed framework incorporates in-depth learning strategies such as CNN architecture and three predefined models called ResNet, InceptionV3. Dataset for seven diseases pictures has been taken to classify skin diseases. Includes diseases such as Melanoma, Nevus, Seborrheic Keratosis etc. The database was expanded by adding cut-and-burn images, classified as skin diseases by most existing systems. The use of Deep Learning algorithms has reduced the need for human activity, such as extracting features and rebuilding data for segmentation purposes.
Exceptional advances in biotechnology and general well-being frameworks have led to significant production of critical and sensitive well-being information. By applying savvy information analysis procedures, many intriguing patterns are recognized for early discovery and anticipation of different lethal illnesses. Diabetes is a very perilous illness since it is related with other deadly infections, i. e. heart, kidney and nerve harm. In this archive a machine learning-based approach has been proposed for the arrangement, early location, and forecast of diabetes. Moreover, a speculative IoT-based diabetes observing framework for a solid and impacted person to screen their blood glucose (BG) levels is introduced. Machine learning classifiers have been utilized to characterize diabetes, with Random Forest (RF), Logistic Regression (LR). Support Vector Machine(SVM), Naive bayes(NB).
EXISTING SYSTEM:-
Over the past few years, the image processing technique as well as the deep convolutional neural networks (CNN) are being widely used for feature extraction and object classification which made it easier to achieve rapid development in the field of medicine. Various scholars all around the world have carried numerous researches on this direction. M. S. AL-Tarawneh [11]studied the problem of Lung cancer detection where the disease was rectified using Image segmentation and feature extraction. C.B. Tatepamulwar, V.P. Pawar, K.S. Deshpande, H. S. Fadewar [7] proposed a diagnosis system based on the techniques of image processing and data mining. Furthermore, Nisha Yadav, Virender Kumar Narang, Utpal Shrivatava [8] proposed a system to detect the diseases like ringworm By using deep learning method, image pattern recognition can be performed automatically after program setting. It can input images into CNN with high accuracy and automatically acquire important features. Therefore, this technique eliminates the need to extract information from images prior to the training process. Flat layers study simple features such as borders within an image. Deeper layers next to the output layer study more complex higher-order functions. The function is being studied. Various researchers, institutions and challenges are working on the automatic diagnosis of skin diseases, and various deep learning methods have been developed to detect dermatological diseases; These have proven themselves in numerous areas. For example, the International Skin Imaging Collaboration (ISIC) is a challenge focused on the automated analysis of skin lesions. The goal of the challenge is to support the research and development of algorithms for automated melanoma diagnosis, including lesion segmentation, detection of dermoscopic features within a lesion, and melanoma classification, which is also the main goal in the field of dermatology.In general, this method is a modeling framework that can learn the functional mapping of input to output images. The input image is a pre-processed image; the output image is a segmentation mask. The network fabric includes a series of convolution and pooling layers, followed by a fully connected layer, followed by a series of unbundling and separating operations.
PROPOSED SYSTEM:
The proposed program aims at automated computer-based diagnostics to reduce life-threatening risks. This has undoubtedly been a challenging task due to the good variety of skin looks.
a holistic approach to diagnosing skin diseases is used to process images and computer diagnostics. In this case the skin images are provided in the system for processing. The inserted image is subject to image processing such as pre-screening, feature removal and a machine-based separator to predict skin disease or not and recommend drug guidance based on the stage of skin disease. The proposed methodology is an effective tool that can process people who develop skin disease to predict skin disease. In this proposed system, multimedia imaging and mechanical learning techniques are used to predict the nature of the disease in a relatively short period of time.
The image processing section requests pre-processing, classification, feature removal steps. The machine learning phase calls for 3 steps: processing steps, training and acquisition. The proposed system uses a 2D Wavelet Transform algorithm to extract the element when color, texture and shape elements are extracted from the input images. Contact values are excluded from the input image. These values are transferred to the division model. To differentiate the proposed system uses the convolutional neural network (CNN). The isolation model finds common skin diseases such as Psoriasis, Lichen Planus, Pityriasis Rosea. The neural network integration provides good precision results. The proposed system serves as the basis for General Disease diagnosis and prescription. This proposed system analyzes a different type of skin disease that can be analyzed to save user time and cost. For the proposed program.
MODULES:
- User Registration: User has to register to check Skin disease and diabetes
- User Login: User can login to system to check Skin disease and diabetes
- CNN model generation: Skin disease categories will be added and trained with convolution neural network with ResNet, InceptionV3 and VGG16.
- Diabetes Detection: Machine learning model trained by user symptoms dataset to classifies that user has diabetes or not.
ADVANTAGES :
In general, the advantage of AI is that it can help doctors perform tedious repetitive tasks. For example, if sufficient blood is scanned, an AI-powered microscope can detect low-density infections in micrographs of standard, field-prepared thick blood films, which is considered to be time-consuming, difficult, and tedious owing to the low density and small parasite size and abundance of similar non-parasite objects . The requirement for staff training and purchase of expensive equipment for creating dermoscopic images can be replaced by software using CNNs . In the future, the clinical application of deep learning for the diagnosis of other diseases can be investigated. Transfer learning could be useful in developing CNN models for relatively rare diseases. Models could also evolve such that they require fewer preprocessing steps. In addition to these topics, a deeper understanding of the reconstruction kernel or image thickness could lead to improved deep learning model performance. Positive effects should continue to grow owing to the emergence of higher precision scanners and image reconstruction techniques . However, we must realize that although AI has the ability to defeat humans in several specific fields, in general the performance of AI is considerably less than acceptable in the majority of cases
HARDWARE REQUIREMENT:-
- Processor – i3,i5
- Hard Disk – 5 GB
- Memory – 6GB RAM
SOFTWARE SPECIFICATION:-
- Windows Operating System.
- MySQL
- Python
- Flask
- Anaconda ,Jupyter, Spyder
APPLICATIONS:
it is clear that the GUI contains many different buttons for different purposes, such as an input image, gray image, preprocessing image, detection of disease, and classification of disease buttons. Hence, for GUI users, direct image uploading into the application can be further used by the system processes. For this reason, the results of each step can be shown on the GUI screen itself as images or displayed in the relevant message box.
Moreover, the first step is to use the input image button to capture an image of the affected skin region. Then, it can be subjected to preprocessing . The second step is to activate the gray image button. Gray image contrast enhancement is performed to improve the clarity of the image, followed by red/green/blue (RGB) to grayscale conversion . The third step is to employ the preprocessing image button, which initializes the performance of two smaller processes. Contrast enhancement is performed using histogram equalization and grayscale conversion. In this process, a raw binary image is transformed into an RGB matrix form to distinguish each pixel from its neighbor. Then, the RGB matrix is converted into a grayscale matrix. The fourth step is to detect the disease . This step involves feature extraction, after which the most convenient details in the image are considered, and other details are discarded. This process is performed to make the detection or classification step much easier.